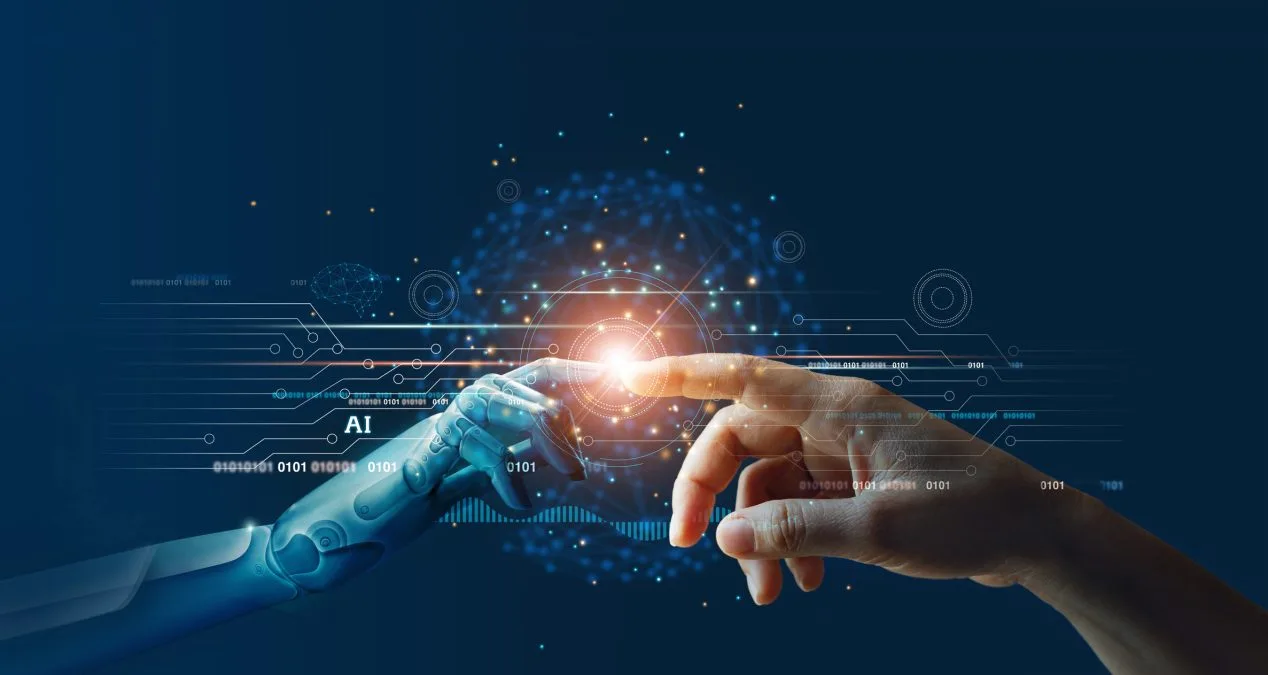
Machine learning, a branch of artificial intelligence, has emerged as a powerful tool in the healthcare domain. It has the potential to revolutionize disease management by enabling accurate predictions of disease outcomes. Traditional medical approaches rely on human expertise and statistical models, which may have limitations in handling complex data. Machine learning algorithms, on the other hand, can analyze vast amounts of data and identify patterns that are difficult for humans to discern. In this article, we explore the application of machine learning in predicting disease outcomes and its potential implications for personalized medicine.
Harnessing the power of data
In the era of big data, healthcare systems generate and store massive amounts of information, including electronic health records, medical imaging data, genomic data, and patient-reported outcomes. Machine learning algorithms can leverage this wealth of data to identify patterns, relationships, and risk factors associated with disease outcomes. By analyzing diverse data sources, these algorithms can generate predictive models that offer valuable insights into disease progression, treatment response, and patient outcomes.
Predictive modeling for disease outcomes
Machine learning algorithms can predict disease outcomes by learning from historical data and patterns. These models can consider a wide range of factors, such as patient demographics, medical history, genetic markers, laboratory results, and treatment interventions. By analyzing these variables collectively, machine learning algorithms can generate accurate predictions regarding disease progression, prognosis, and treatment response.
One of the significant advantages of machine learning models is their ability to handle complex, non-linear relationships and interactions between various factors. Traditional statistical models may struggle to capture these intricate connections, but machine learning algorithms excel in identifying subtle patterns and associations within the data.
Applications in personalized medicine
Predicting disease outcomes through machine learning has immense implications for personalized medicine. By analyzing individual patient data, including genetic information, lifestyle factors, and medical history, machine learning algorithms can generate personalized risk profiles. These profiles can help healthcare providers tailor treatments and interventions specifically to each patient, optimizing outcomes and minimizing adverse effects.
Machine learning can also assist in treatment selection and optimization. By analyzing data on treatment responses and outcomes from a large cohort of patients, algorithms can identify patterns and factors associated with treatment success or failure. This information can guide clinicians in selecting the most effective treatment options for individual patients, improving the chances of positive outcomes.
Challenges and ethical considerations
While machine learning shows tremendous promise in predicting disease outcomes, certain challenges and ethical considerations need to be addressed. One challenge is the quality and availability of data. Machine learning algorithms heavily rely on high-quality, diverse, and well-curated datasets. Incomplete or biased data can result in inaccurate predictions or reinforce existing disparities in healthcare.
Another concern is the interpretability and transparency of machine learning models. The black-box nature of some algorithms makes it challenging to understand the underlying factors contributing to predictions. Explainable AI techniques are being developed to address this issue, allowing clinicians and patients to trust and understand the decision-making process of these algorithms.
Additionally, privacy and security of patient data must be ensured. Proper safeguards should be in place to protect sensitive medical information and maintain patient confidentiality while enabling data sharing for research purposes.
Machine learning has the potential to revolutionize disease management by accurately predicting disease outcomes. By leveraging large-scale data analysis and pattern recognition, machine learning algorithms can provide personalized risk profiles, treatment recommendations, and prognostic insights. However, challenges related to data quality, interpretability, and privacy must be addressed to ensure the responsible and ethical implementation of these algorithms in healthcare. As machine learning continues to advance, it holds the promise of transforming medicine by enabling more precise and personalized care for patients.