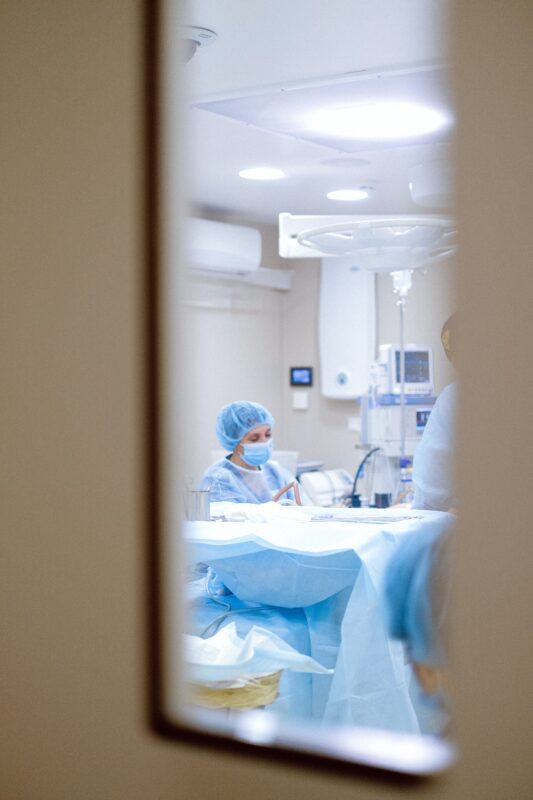
The field of healthcare is experiencing a transformative revolution, thanks to the integration of machine learning (ML) techniques. With the ability to analyze vast amounts of data, ML algorithms have the potential to revolutionize early disease detection. By leveraging this technology, healthcare professionals can identify patterns and subtle indicators of diseases at their earliest stages, enabling timely interventions and improved patient outcomes. In this article, we explore the remarkable potential of ML in early disease detection and its impact on the future of healthcare.
Unleashing the potential of big data,
One of the key advantages of ML in early disease detection is its ability to process and analyze large volumes of data. Electronic health records, medical imaging, genomic data, and wearable sensor data generate massive amounts of information. ML algorithms can uncover hidden patterns, correlations, and trends within this data that may indicate the presence of diseases, even before clinical symptoms manifest. By integrating and analyzing diverse datasets, ML algorithms can learn to recognize complex patterns that are difficult for human observers to identify, leading to more accurate and timely diagnoses.
Early detection for improved outcomes,
Early disease detection is crucial for successful treatment and improved patient outcomes. Many diseases, such as cancer, cardiovascular conditions, and neurodegenerative disorders, are most treatable in their early stages. ML algorithms can assist in identifying subtle deviations from normal patterns that indicate the presence of disease. By analyzing patient data over time, ML algorithms can learn to recognize early warning signs and flag individuals who may be at risk. This enables healthcare providers to intervene promptly, providing personalized treatment plans and reducing the progression of the disease.
Predictive analytics for risk assessment,
Machine learning excels in predictive analytics, providing valuable insights into an individual’s risk of developing certain diseases. By training on large datasets of patient records, ML algorithms can identify risk factors and predict the likelihood of disease occurrence. These algorithms consider a variety of factors, such as demographics, genetic markers, lifestyle choices, and medical history, to generate risk scores for individuals. This information can help healthcare providers implement preventive measures, such as lifestyle interventions, targeted screenings, and early interventions, reducing the burden of disease and improving population health.
Improving medical imaging and diagnostics,
Medical imaging plays a critical role in disease detection, and ML algorithms have shown tremendous promise in improving accuracy and efficiency in this domain. By analyzing large datasets of medical images, ML algorithms can learn to detect subtle patterns and anomalies that may indicate the presence of diseases, such as tumors or abnormalities in organ structures. These algorithms can aid radiologists in their assessments, providing a second set of “virtual eyes” and reducing the chances of human error. ML-powered diagnostic systems have the potential to speed up diagnoses, streamline workflows, and improve the accuracy of interpretations, ultimately benefiting patients and healthcare providers alike.
Challenges and ethical considerations,
While ML holds immense potential, there are challenges and ethical considerations that must be addressed. ML algorithms heavily rely on high-quality and diverse datasets for training. Ensuring data privacy, maintaining data integrity, and addressing biases in the data are critical concerns. Additionally, the interpretability and transparency of ML algorithms are essential to gain the trust of healthcare professionals and patients. A balance between innovation and accountability must be struck to ensure that ML algorithms are reliable, safe, and equitable in their application.
The future of early disease detection,
The future of early disease detection is incredibly promising with the integration of ML technologies. As ML algorithms continue to evolve, they will become more accurate, efficient, and accessible. Combining ML with emerging technologies like wearable devices and real-time monitoring systems can create a continuous feedback loop, providing early detection and personalized interventions in real-time. Additionally, collaborations between researchers, clinicians, and data scientists are essential to drive innovation and overcome challenges in implementing ML-based early disease detection systems.
Machine learning is transforming healthcare by revolutionizing early disease detection. By leveraging the power of ML algorithms to analyze vast amounts of data, healthcare professionals can identify patterns, predict risks, and detect diseases at their earliest stages. This technology has the potential to improve patient outcomes, reduce healthcare costs, and enhance population health. While challenges exist, continued research, innovation, and ethical considerations will pave the way for a future where early disease detection becomes a routine part of healthcare, benefiting individuals and societies worldwide.